Plenary
Lecture
Computational Methods In Real Life Problem
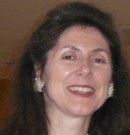
Assossiate Professor
Alina Barbulescu
Ovidius University of Constanta,
Faculty of Mathematics and Computers Science,
Constanta, ROMANIA
E-mail: abarbulescu@univ-ovidius.ro
Abstract:
The time series analysis is an ample domain of study,
implying multiple approaches, in time and in frequency
domain. The difficulties that appear in the modeling of
the non - stationary time series are, essentially: the
noise presence, the elaboration of the techniques of
noise estimation and removal, the perturbations
detection and measurement, the long dependence in the
data series and the errors propagation. There is also
the question on the accuracy of the entrance data.
Generally, the models from the nature sciences have a
deterministic a stochastic component. The pure
stochastic models are used if the causality relations of
the phenomena are not known. The pure deterministic ones
are developed especially in meteorology and try to
reproduce the dynamic of the rainfall field, based on
Navier-Stokes equations, blunted, approximated and then
numerical integrated (in the hypothesis of scale
homogeneity). In spite of simplifying, the resulted
equations remain complex, the calculation is difficult
and the scales are independently studied one to others.
So, the algorithm and the calculus methodology must be
improved.
There is the tendency to work in restrictive hypotheses
on the data (stationarity, homoscedasticity,
independence etc.) or one tries to bring them in
standard form, by different transformations. But, in
majority, the in nature sciences, the series are not
stationary and heteroscedastic, needing decomposition
procedures, to be modeled. In plus, they follow varied
statistical laws and the data are not independent,
presenting usually a long or short dependence in time.
In our article we shall discuss this problems and their
computational solution in modeling the time series, from
classical ones (decomposition methods, deterministic
models and stochastic models), to modern ones (GEP,
AdaGEP and nonparametric), with concrete case study from
meteorological time series.
Brief Biography of the Speaker:
Alina Barbulescu graduated from the University of
Craiova, Romania (Mathematics) and from Petre Andrei
University of Iasi, Romania (Faculty of Law). After a
PhD in Mathematics, from Al I Cuza University of Iasi
and one in Cybernetics and Economic Statistics, from
Academy of Economic Studies Bucharest, Romania, she
worked in the field of mathematice and applied
statistics. Nowadays she is associate professor at
Ovidius University of Constanta, faculty of mathematics
and Computer Science. She is author of 18 books and over
90 articles, published in peer rewieved international
journal, being also a member of editorial boards of
International Journal of Mathematics and Computation and
International Journal of Applied Mathematics and
Statistics.
|