Keynote Lecture
Optimization Based Matrix Decomposition Methods and their Utilization in
Applications
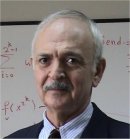
Professor Metin Demiralp
Informatics Institute
Istanbul Technical University
ITU Bilisim Enstitusu Ayazaga Yerleskesi
Maslak, 34469, Istanbul, Turkey
E-mail: metin.demiralp@gmail.com
Abstract: Matrix decomposition methods
play important roles in the theoretical aspects of the matrix theory beside
their utilization in approximations. The main purpose is to represent a
matrix in terms of the rather simple matrices. The linear combination type
representations are mostly preferred to get the benefits of the linearity.
The simple matrices in the linear combination are chosen in as lower ranks
as possible. The one-rank matrices or in other words outer products are
mostly preferred ones. For example, spectral decompositions use outer
products constructed as the product of each normalized eigenvector of the
considered matrix by its transpose or hermitian conjugate and the linear
combination coefficients are the corresponding eigenvalues when the symmetry
or the hermiticity exists in the matrix under consideration. If the symmetry
or hermiticity does not exist then the cases should be investigated
separately for two different categories first of which involves the matrices
with eigenvalues whose algebraic and geometric multiplicities are same while
the second one covers the matrices at least one of whose eigenvalues has
different algebraic and geometric multiplicities. The first group matrices
have spectral decompositions almost same as the symmetric or hermitian
matrices with the only difference in the construction of the outer products
which are now constructed as the product of the right eigenvectors by their
companion transposed left ones. Although the individual normalizations of
the left and right eigenvectors are not necessary the mutual normalizations
are required to give unit norm to each outer product. The second group
matrices can not be expressed in the abovementioned form of spectral
decompositions because they can not be diagonalized. Hence, their
reducibility to Jordan canonical form must be reflected to the
decomposition. What we have stated above is for square matrices. The similar
decomposition for the rectangular matrices is based on the idea of the
forward and backward transitions between two different dimensional Euclidean
spaces. The result is called singular value decomposition where the outer
products are constructed from the left and right singular vectors while the
linear combination coefficients of the decomposition are the singular values
of the matrices which are in fact the square root of the eigenvalues of the
product of the transposed form of the matrix by itself. All these
decompositions can be connected to the optimization theory by defining
appropriate cost functionals and constraints. The cost functional has
quadratic natures in general. The constraints may also be quadratic although
the bilinear forms are encountered as well. The structure of the cost
functional uniquely defines the characters of decomposition. It is possible
to define new and more general decompositions by changing the structures of
the cost functional and the constraints. There have been certain efforts to
do so in recent years. The author and his colleagues are attempting to
construct new schemes to decompose matrices and to use them in modern
applications related to data processing. The talk will focus on the issue in
a more general perspective and try to adress to the works by emphasizing on
the recent ones from and outside the group of the author.
Brief Biography of the Speaker:
Metin Demiralp was born in Turkey on 4 May 1948. His education from
elementary school to university was entirely in Turkey. He got his BS, MS,
and PhD from the same institution, Istanbul Technical University. He was
originally chemical engineer, however, through theoretical chemistry,
applied mathematics, and computational science years he was mostly working
on methodology for computational sciences and he is continuing to do so. He
has a group (Group for Science and Methods of Computing) in Informatics
Institute of Istanbul Technical University (he is the founder of this
institute). He collaborated with the Prof. Herschel A. Rabitz’s group at
Princeton University (NJ, USA) at summer and winter semester breaks during
the period 1985–2003 after his 14 months long postdoctoral visit to the same
group in 1979–1980.
Metin Demiralp has more than 70 papers in well known and prestigious
scientific journals, and, more than 110 contributions to the proceedings of
various international conferences. He has given many invited talks in
various prestigious scientific meetings and academic institutions. He has a
good scientific reputation in his country and he is the full member of
Turkish Academy of Sciences since 1994. He is also a member of European
Mathematical Society and the chief–editor of WSEAS Transactions on
Mathematics currently. He has also two important awards of Turkish
scientific establishments.
The important recent focii in research areas of Metin Demiralp can be
roughly listed as follows: Fluctuation Free Matrix Representations, High
Dimensional Model Representations, Space Extension Methods, Data Processing
via Multivariate Analytical Tools, Multivariate Numerical Integration via
New Efficient Approaches, Matrix Decompositions, Quantum Optimal Control.